Rigorous economic modeling of food systems, designed to inform decision-makers on the impacts of potential policy choices, is central to IFPRI’s work.
IFPRI-led modeling systems assess how different policies and investments affect nutrition, poverty, social inclusion, climate change, and environmental health. These models can incorporate real-time events and test future scenarios based on varying social and climate trends. They operate across different time scales and geographic scales, from local to global, and cover everything from specific agricultural sectors to entire economies.
In this webinar series, our researchers present insights from IFPRI’s key modeling systems and their outputs, developed with other CGIAR Centers and partners. This work is helping to answer the critical questions facing decision-makers and stakeholders in today’s agrifood systems: What does climate change mean for the future of agriculture? How do we prioritize different agrifood system policies and investments? What are the sources, impacts, and trade-offs of agricultural productivity growth? What policy steps should governments take when a crisis strikes and a rapid response is required?
Recent in this series
-
How Can We Improve Food Security Monitoring in Conflict-Affected Regions? Machine Learning for Spatially Granular Food Security Mapping
-
How can we improve global crop mapping? IFPRI’s Spatial Production Allocation Model (SPAM)
-
How should governments respond to crises? Rapid response using RIAPA modeling system
-
How does agricultural productivity growth affect agrifood system transformation goals? Exploring trade-offs using IMPACT

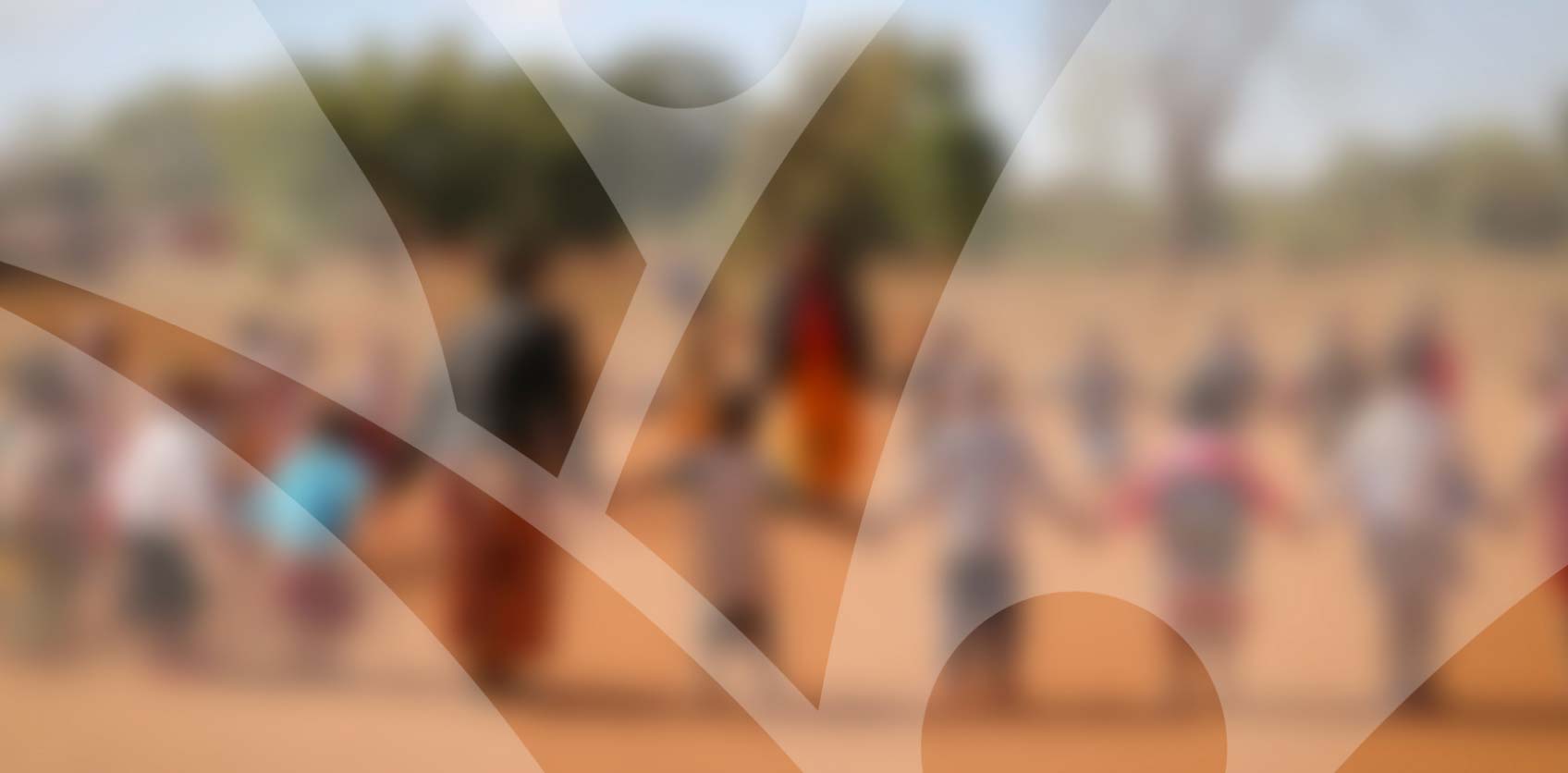
In this seminar, Zhe Guo and Joanna van Asselt explored how machine learning is transforming agricultural and food security research. They presented innovative work using machine learning to estimate Food Consumption Scores at the village-tract level—an approach that combines phone surveys, earth observation, crowd-sourced data, and GIS to produce timely, localized food security assessments. The discussion covers key technical aspects of this work, including data integration, feature selection, and model validation, as well as the broader potential for scaling up this method in fragile and conflict-affected contexts to inform humanitarian and policy responses.